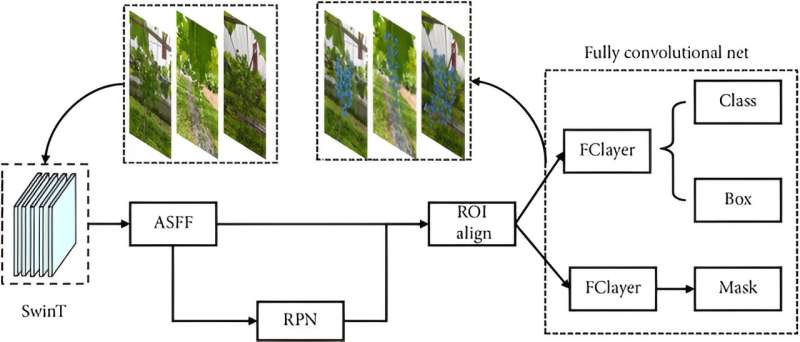
Berry thinning is crucial for cultivating high-quality table grapes, traditionally carried out by skilled laborers who are becoming scarce due to an aging population. To address the labor-intensive and time-consuming process, researchers are focused on developing an intelligent machine vision system for automated berry thinning.
Current studies utilize deep learning (DL) and image processing to count and locate berries, with methodologies ranging from analyzing whole vine images to focusing on single-grape-bunch images for improved accuracy and efficiency. However, challenges persist in accurately detecting small berries during the thinning season due to their size, color similarity to leaves, and dense packing. This necessitates further research to enhance detection capabilities and generalize the techniques.
In this study, the researchers employed a machine vision system, specifically an instance segmentation model named AS-SwinT, to enhance the process of grape berry counting. The experiment was conducted using a robust hardware setup, including a 24GB NVIDIA RTX3090 and i7 CPU. Software tools such as Python and PyTorch were utilized, employing MMDetection for model construction.
Images were enhanced through flipping techniques, and the models were trained using AdamW optimization, with a focus on transfer learning and backbone weights from the Imagenet dataset. The performance of AS-SwinT and other models was evaluated using COCO-style average precision metrics, considering various object sizes and employing precision and recall calculations.
Ablation studies revealed significant improvements in detection accuracy, especially with the integration of Anchor optimization, Soft-NMS, and ASFF components. AS-SwinT demonstrated superior performance in detecting small berries, combining the advantages of these modules to avoid miss detection.
Comparisons with different backbone networks showed that deepening layers don’t always correlate with higher accuracy, highlighting the Swin Transformer’s effective feature extraction capability. When compared with classical models in both artificial and natural environments, AS-SwinT outperformed others, showing remarkable robustness and accuracy, especially in challenging conditions where berries overlap or are shaded.
The study also explored the number estimation of berries in a single bunch using a linear regression model, assessing the relationship between prediction and ground truth. AS-SwinT achieved the highest fitting characteristics among the models tested, indicating its potential utility for grape growers.
Despite the success, challenges like the non-detection of heavily shaded or tiny berries highlight areas for future research, including the exploration of different grape varieties and the impact of environmental conditions on detection accuracy. The study aims to reduce labeling costs and improve the model further.
It suggests that semi-supervised learning and other methods could be beneficial. Overall, the research presents a significant advancement in automated grape berry counting, offering a robust solution to assist in the berry-thinning process and potentially revolutionizing table grape cultivation.
More information:
Wensheng Du et al, Instance Segmentation and Berry Counting of Table Grape before Thinning Based on AS-SwinT, Plant Phenomics (2023). DOI: 10.34133/plantphenomics.0085
Citation:
Revolutionizing grape cultivation: AS-SwinT and the future of automated berry thinning (2023, December 29)
retrieved 31 December 2023
from https://phys.org/news/2023-12-revolutionizing-grape-cultivation-as-swint-future.html
This document is subject to copyright. Apart from any fair dealing for the purpose of private study or research, no
part may be reproduced without the written permission. The content is provided for information purposes only.