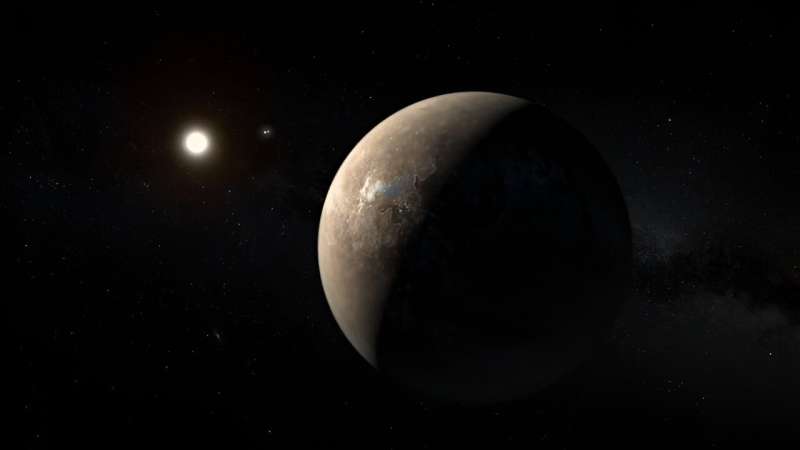
How can machine learning help astronomers find Earth-like exoplanets? This is what a new study hopes to address as a team of international researchers investigated how a novel neural network-based algorithm could be used to detect Earth-like exoplanets using data from the radial velocity (RV) detection method.
This study holds the potential to help astronomers develop more efficient methods in detecting Earth-like exoplanets, which are traditionally difficult to identify within RV data due to intense stellar activity from the host star. The study is published on the arXiv preprint server.
The study notes, “Machine learning is one of the most efficient and successful tools to handle large amounts of data in the scientific field. Many algorithms based on machine learning have been proposed to mitigate stellar activity to better detect low-mass and/or long period planets. These algorithms can be classified into two categories: supervised learning and unsupervised learning. The advantage of supervised learning is that the proposed model contains a large set of variables and has the ability to produce relatively accurate predictions based on the training data.”
For the study, the researchers applied their algorithm to three stars to ascertain its ability to identify exoplanets within the stellar activity data: our sun, Alpha Centauri B (HD 128621), and Tau ceti (HD 10700), with Alpha Centauri B being located approximately 4.3 light-years from Earth and Tau ceti being located approximately 12 light-years from Earth.
After inserting simulated planetary signals within the algorithm, the researchers found their algorithm successfully identified simulated exoplanets with potential orbital periods ranging between 10 to 550 days for our sun, 10 to 300 days for Alpha Centauri B, and 10 to 350 days for Tau ceti.
It’s important to note that Alpha Centauri B currently has had several potential exoplanet detections but none confirmed while Tau ceti currently has eight exoplanets listed as “unconfirmed” within its system.
Additionally, the algorithm identified these results correspond to Alpha Centauri B and Tau ceti potentially having exoplanets approximately four-times the size of Earth and within the habitable zones of those stars, as well. After inserting more stellar activity data into the algorithm, the researchers discovered the algorithm successfully identified a simulated exoplanet approximately 2.2-times the size of the Earth while orbiting the same distance as the Earth from our sun.
The study noted in its conclusions, “In this paper, we developed a neural network framework to efficiently mitigate stellar activity at the spectral level, to enhance the detection of low-mass planets on periods from a few days up to a few hundred days, corresponding to the habitable zone of solar-type stars.”
While the study focused on finding Earth-like exoplanets within RV data, the researchers note that additional data, including transit time, phase, and space-based photometry, could be used to identify Earth-like exoplanets.
They emphasize the European Space Agency’s PLATO space telescope mission could accomplish this, which is currently being developed and slated for launch sometime in 2026. Upon launch, it will be stationed at the sun-Earth L2 Lagrange point located on the opposite side of the Earth from the sun where it will scan up to one million stars searching for exoplanets using the transit method with an emphasis on terrestrial (rocky) exoplanets.
This study comes as the number of confirmed exoplanets by NASA has reached 5,632 as of this writing, which is comprised of 201 terrestrial exoplanets, and also provides the upcoming PLATO mission ample opportunity to discover many more terrestrial exoplanets within our Milky Way galaxy.
More information:
Yinan Zhao et al, Improving Earth-like planet detection in radial velocity using deep learning, arXiv (2024). DOI: 10.48550/arxiv.2405.13247
Citation:
A new deep-learning algorithm can find Earth 2.0 (2024, May 31)
retrieved 2 June 2024
from https://phys.org/news/2024-05-deep-algorithm-earth.html
This document is subject to copyright. Apart from any fair dealing for the purpose of private study or research, no
part may be reproduced without the written permission. The content is provided for information purposes only.